1CISPA Helmholtz Center for Information Security
2Warsaw University of Technology
3IDEAS NCBR
*Indicates Equal Contribution
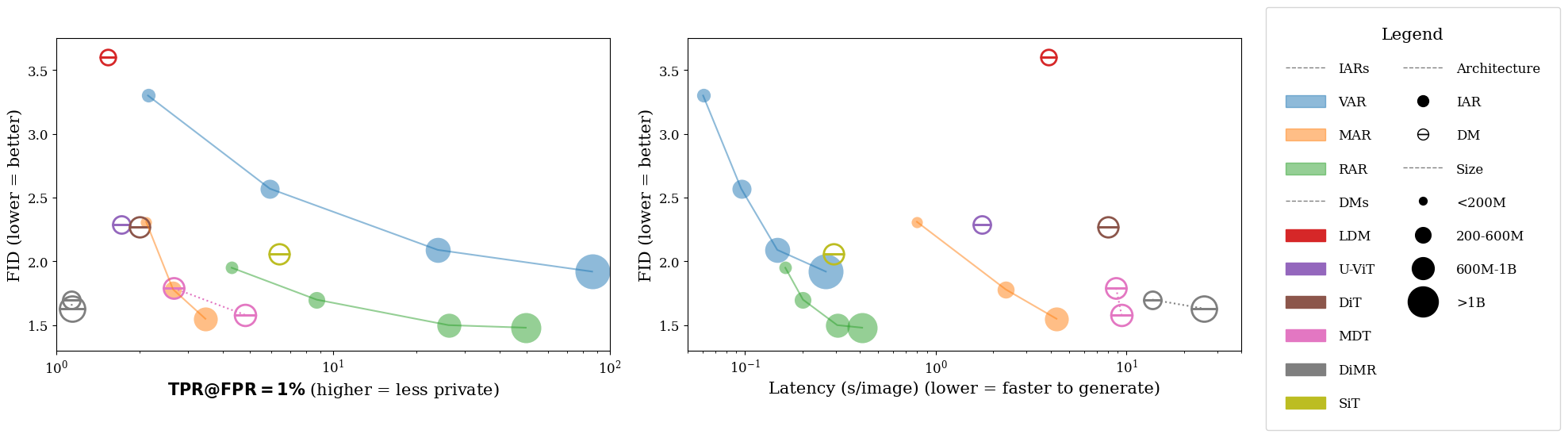
Abstract
Image AutoRegressive generation has emerged as a new powerful paradigm with
image autoregressive models (IARs) surpassing state-of-the-art diffusion models
(DMs) in both image quality (FID: 1.48 vs. 1.58) and generation speed. However,
the privacy risks associated with IARs remain unexplored, raising concerns regard-
ing their responsible deployment. To address this gap, we conduct a comprehensive
privacy analysis of IARs, comparing their privacy risks to the ones of DMs as
reference points. Concretely, we develop a novel membership inference attack
(MIA) that achieves a remarkably high success rate in detecting training images
(with a TPR@FPR=1% of 86.38% vs. 4.91% for DMs with comparable attacks).
We leverage our novel MIA to provide dataset inference (DI) for IARs, and show
that it requires as few as 6 samples to detect dataset membership (compared to
200 for DI in DMs), confirming a higher information leakage in IARs. Finally, we
are able to extract hundreds of training data points from an IAR (e.g., 698 from
VAR-d30). Our results demonstrate a fundamental privacy-utility trade-off: while
IARs excel in image generation quality and speed, they are significantly more
vulnerable to privacy attacks compared to DMs. This trend suggests that utilizing
techniques from DMs within IARs, such as modeling the per-token probability
distribution using a diffusion procedure, holds potential to help mitigating IARs’
vulnerability to privacy attacks.
Contributions
- Our new MIA for IARs achieves extremely strong performance of even 86.38% TPR@FPR, improving over naive application of MIAs by up to 69%.
- We provide a potent DI method for IARs, which requires as few as 6 samples to assess dataset membership signal.
- We propose an efficient method of training data extraction from IARs, and successfully extract up to 698 images.
- IARs outperform DMs in generation efficiency and quality but suffer order-of-magnitude higher privacy leakage compared to them in MIAs, DI, and data extraction.
Membership Inference Attacks Results
Model | VAR-d16 | VAR-d20 | VAR-d24 | VAR-d30 | MAR-B | MAR-L | MAR-H | RAR-B | RAR-L | RAR-XL | RAR-XXL |
---|---|---|---|---|---|---|---|---|---|---|---|
Baselines | 1.62 | 2.21 | 3.72 | 16.68 | 1.69 | 1.89 | 2.18 | 2.36 | 3.25 | 6.27 | 14.62 |
Our Methods | 2.16 | 5.95 | 24.03 | 86.38 | 2.09 | 2.61 | 3.40 | 4.30 | 8.66 | 26.14 | 49.80 |
Improvement | +0.54 | +3.73 | +20.30 | +69.69 | +0.40 | +0.73 | +1.22 | +1.94 | +5.41 | +19.87 | +35.17 |
We improve over baseline Membership Inference Attacks by up to 69.69% for VAR-d30.
Dataset Inference Results
Model | VAR-d16 | VAR-d20 | VAR-d24 | VAR-d30 | MAR-B | MAR-L | MAR-H | RAR-B | RAR-L | RAR-XL | RAR-XXL |
---|---|---|---|---|---|---|---|---|---|---|---|
Baseline | 2000 | 300 | 60 | 20 | 5000 | 2000 | 900 | 500 | 200 | 40 | 30 |
+Optimized Procedure | 600 | 200 | 40 | 8 | 4000 | 2000 | 800 | 300 | 80 | 30 | 10 |
Improvement | -1400 | -100 | -20 | -12 | -1000 | 0 | -100 | -200 | -120 | -10 | -20 |
+Our MIAs for IARs | 200 | 40 | 20 | 6 | 2000 | 600 | 300 | 80 | 30 | 20 | 8 |
Improvement | -400 | -160 | -20 | -2 | -2000 | -1400 | -500 | -220 | -50 | -10 | -2 |
Data Extraction
We successfully perform data extraction attack against IARs, extracting up to 698 images. We extract 698 images for VAR-d30, 5 images for MAR-H, and 36 images for RAR-XXL.
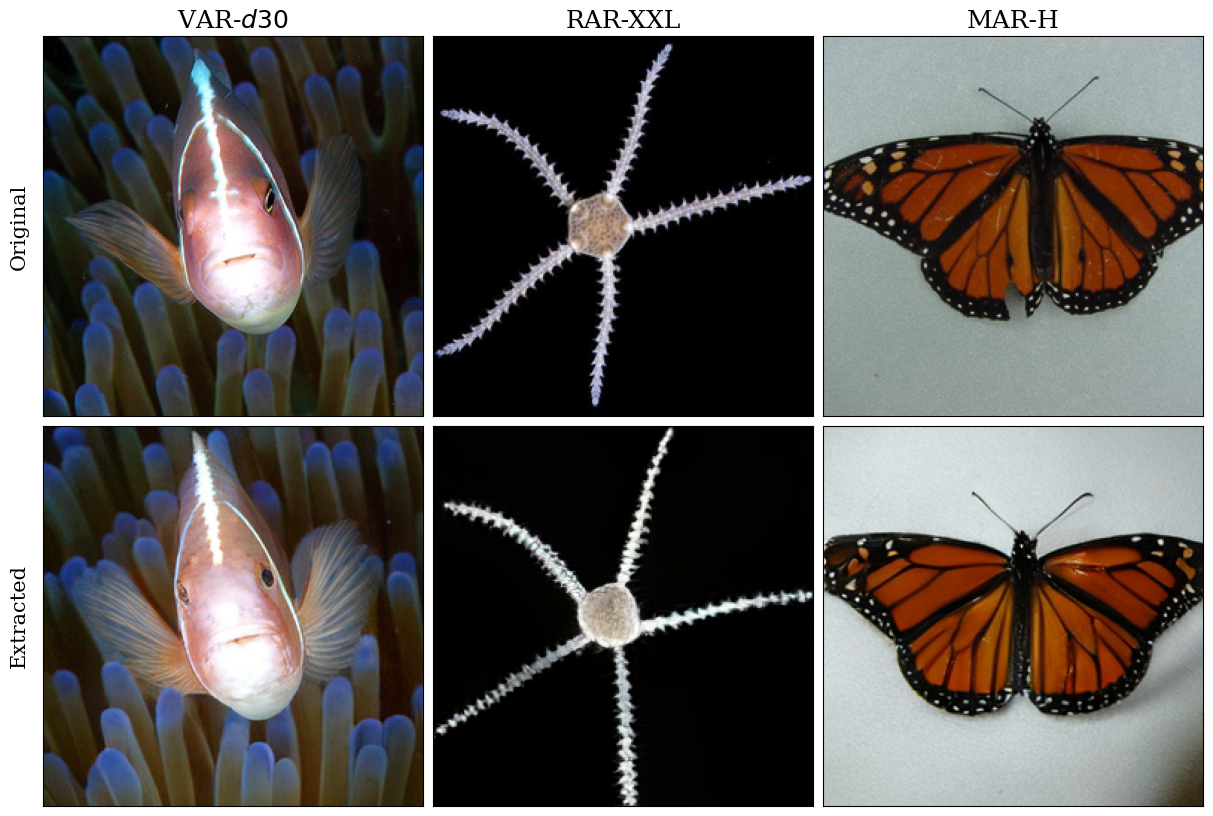
Bibtex
@misc{kowalczuk2025privacyattacksimageautoregressive,
title={Privacy Attacks on Image AutoRegressive Models},
author={Antoni Kowalczuk and Jan Dubiński and Franziska Boenisch and Adam Dziedzic},
year={2025},
eprint={2502.02514},
archivePrefix={arXiv},
primaryClass={cs.CV},
url={https://arxiv.org/abs/2502.02514},
}